Chris Wiggins, the theoretical physicist working at the New York Times
When Chris Wiggins says he’s working as a data scientist at the New York Times, most people think he’s crafting interactive data visualizations or even masterminding the future of journalism. But the real reason the New York Times hired a theoretical physicist is to explore, with scientific precision, the real-time data being generated by the millions of people who interact with the newspaper’s content every day.
Like any newspaper, the New York Times is always trying to upgrade its readers to the next level. They want casual visitors to become loyal visitors, and loyal visitors to become subscribers. But the New York Times doesn’t have dossiers on its millions of readers that would reveal their reading and purchasing habits.
“What you are armed with is a bunch of data about [each reader],” he told an auditorium packed with computer and natural scientists at Harvard University recently. “By scrolling and clicking you are giving the New York Times information.” Creeped out? Given the amount of big data being collected by everyone from retailers to the NSA, the Times is the least of your problems. But of course the real art and craft behind big data isn’t the quantity, but the quality of your analysis. That’s where Wiggins comes in:
It’s his mission to put the pieces together.
Using various analytics engines, advertising services and cookies–some of which won’t expire for years–the NYTimes knows:
- How you arrived at a page
- Whether you’re a new user or logged in
- How long you spend on a page
- How you scroll down the page
- Which story you’re hovering over
- How many stories you read in a session
- Whether you are getting any error messages
- How ads will be served to you
- Various other behavioral metrics that reflect how you experience and interact with the New York Times website
Using these metrics, Wiggins builds models to predict not only what a New York Times reader does but whether she will be there tomorrow.
Wiggins calls it computational social science. In essence, he says, he is running a randomized clinical trial—day in and day out—wherein he is predicting user response. In this case, he’s not measuring therapeutic benefit (or adverse side effects) from a drug but rather loyalty to (or attrition from) the NYTimes product.
The funnel
He calls this the ‘funnel’ and—like it or not—it applies to every company in journalism. Given the metrics you measure about your audience, can you predict how many frequent readers will become loyal subscribers, how many infrequent readers will become frequent readers, and how many infrequent readers will stop coming all together?
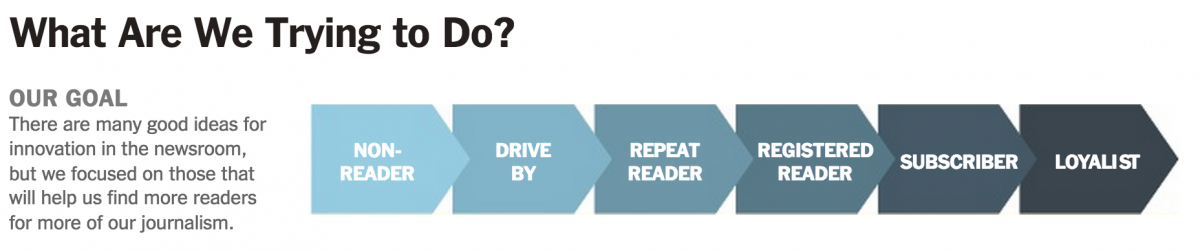
Wiggins and his team at the New York Times started off developing a probability model, where millions of readers were categorized into discrete clusterable groups. More information about statistical classification here. Tweaking the composition of those groups yields different conclusions about their behavior.
Data in your newsroom
Wiggins underscores the need to understand several things about data before a newsroom goes hog wild hiring data scientists. [inlinetweet prefix=”” tweeter=”” suffix=” says @chrishwiggins”]“Don’t hire a data scientist if you haven’t built the pipes yet,”[/inlinetweet] he warned, meaning that [inlinetweet prefix=”” tweeter=”” suffix=” via @story_bench”]to leverage data properly, engineers must do a lot of programmatic pipe-building[/inlinetweet] to access that data and make it malleable enough to do something with. An outlet can’t just open the firehose without building pipes to route that information.
Once you’ve laid those pipes—in the form of APIs and language (such as JSON) with which to output data—only then can you get to the step of interpreting what’s coming through it. That’s when visualization and computational analysis come into play.
Three points on data literacy
Those you’ve staffed to fill these data engineer and data scientist positions should be able to do the following, Wiggins says.
- Demonstrate rhetorical literacy (i.e. be able to explain the fancy chart they’ve made)
- Demonstrate critical literacy (i.e. be skeptical of data and aware of cherry-picking)
- Share tools, empower others with data-savviness