How news media are setting the 2020 election agenda: Chasing daily controversies, often burying policy
It’s a paradox of examining political coverage. Are news media just reporting what the political candidates are talking about? Or does political journalism really set the agenda by selecting stories around specific news items, scandals and issues du jour?
Our topic analysis of ~10,000 news articles on the 2020 Democratic candidates, published between March and October in an ideological diverse range of 28 news outlets, reveals that political coverage, at least this cycle, tracks with the ebbs and flows of scandals, viral moments and news items, from accusations of Joe Biden’s inappropriate behavior towards women to President Trump’s phone call with Ukraine. (A big thanks to Media Cloud.)
This tendency, in turn, allows important issues such as health care, climate change and reproductive rights to fall off the agenda every time a Trump-driven media cycle emerges from some new outrage or a flavor-of-the-day controversy pops up. The following are some of our findings (more on our methods at the bottom):
- Coverage of the candidates’ positions on immigration and health care flares up during the debates but quickly subsides.
- Since Kirsten Gillibrand dropped out, no candidate has picked up the topic of reproductive rights, at least in terms of what the media is reporting.
- The guns topic has been most prevalent in O’Rourke coverage, thanks to his response to the El Paso shooting and later his viral moment during the September debate. Earlier this month O’Rourke ended his campaign.
- The Ukraine topic has dominated recent coverage of the 2020 candidates and is most closely associated with coverage of Biden.
- The impeachment topic, which includes Attorney General Bill Barr, former special counsel Robert Mueller and Russia, has waned during debate coverage but is now firmly back on the agenda.
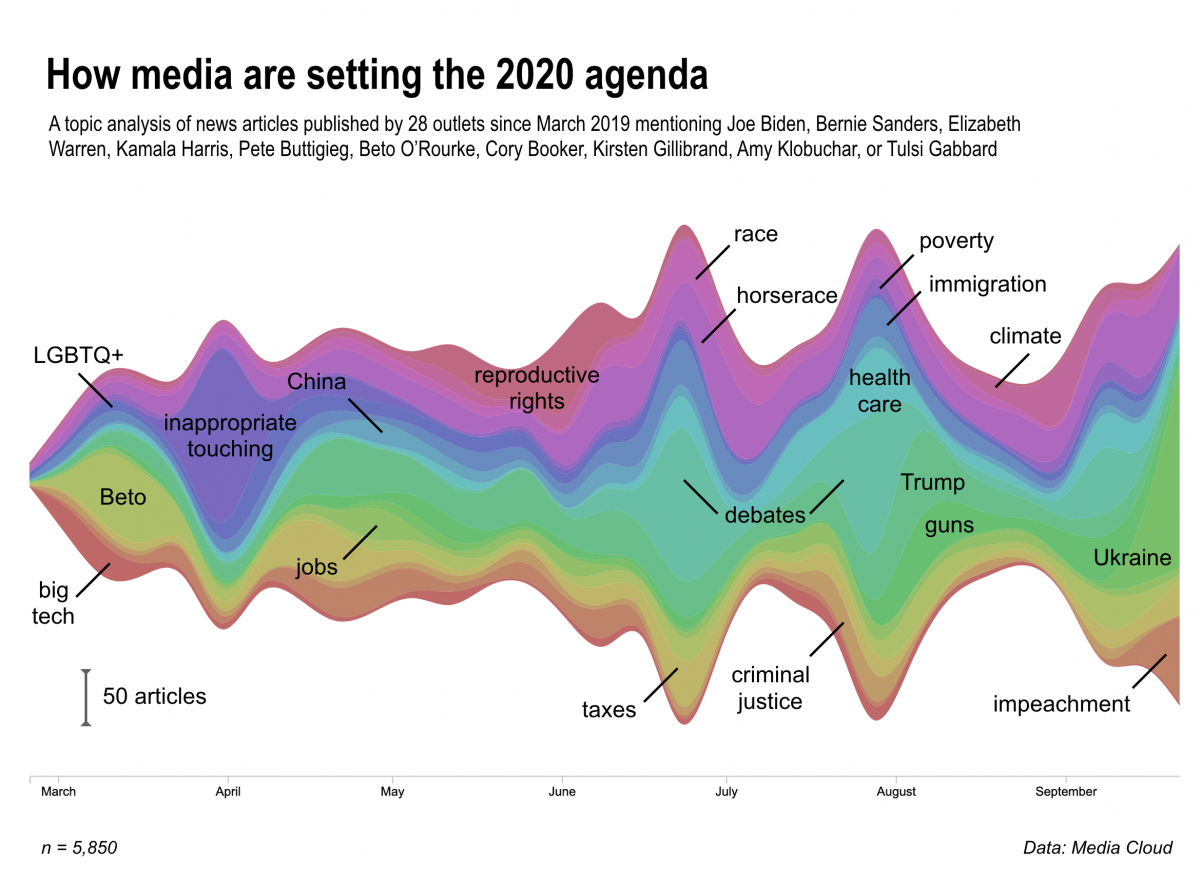
The colorful stream graph above represents 20 highly prevalent and highly coherent topics that emerge from news media coverage over this period (we tossed out clusters of common/generic campaign words; see our code at bottom of this post). Yet it is instructive to consider the next five topics down in our rough ranking order, none of which are in the graphic: sexual harassment and gender issues; voting rights; child poverty and economic opportunity; health care and affordability; education. All of these are core Democratic Party issues, but each is buried below at least 20 other trending topics over the course of the campaign so far; and often substantive issues wholly disappear when a big controversy breaks out.
It’s worth putting in larger context the Rorschach-like blob that is the media agenda this year: Pundits often say the Republican Party is in disarray, but based on this analysis of media coverage of Democratic candidates in 2019, the average U.S. voter is unlikely to be clear yet what Democrats want this election to be about. This may simply seem the messiness of democracy at work — let a thousand campaign fights, flare-ups, and ideas bloom — but it’s probably far from ideal if you are the Democratic Party chair. Political scientists and campaign practitioners alike agree that you must repeat messages over and over, for long periods, for anything durable to sink in with the public.
The diffuse nature of the media agenda may of course be a function of the large Democratic field of candidates, a fact that does not lend itself to easy, repeated topics for coverage and more neatly defined media narratives. In this election cycle, news media are simply all over the place, often moving in tandem to chase the latest trend.
For several generations now, most media coverage of presidential politics has consisted of pack journalism focused on polls and the controversies of the day. Given the press’ disastrous performance in the 2016 campaign, one might have hoped that it would be different this time around.
So far, though, it’s been business as usual, which our analysis helps confirm.
Unpacking the data
While automated large-scale content analysis is prone to false positives and false negatives and should not replace close reading of text, as researchers have been careful to point out, these kinds of methods are increasingly becoming a standard tool for political and social scientists, and data journalists, too. Similar topic modeling has been applied to Senate speeches to infer political attention, Senate press releases to measure political agendas, and Congressional statements to reveal framing and agenda setting.
Inherently, there will be mislabeling with topic modeling, as this seminal paper discusses, and we’ve tried to address this by removing poorly-defined topics from the final analysis. Of the 60 topics we initially assigned to the 9,854 articles published in 28 media outlets since March, we selected 20 that were highly prevalent in the dataset and highly coherent as topics, bringing the total to 5,850 articles, and visualized the weekly number of articles most closely related to those topics.
While these appear to be the clearest “signals” that we can discern, using both computational and human review methods, emerging from the noise of the campaign coverage, there are, of course, caveats. The ranking and clustering of individual topics are based on estimates. Yet the overall picture is likely accurate.
Click here to view a full-screen, interactive version of the following visualization, which plots the 5,850 articles where these 20 topics are most prevalent. Clicking on each dot will bring you to the news article.
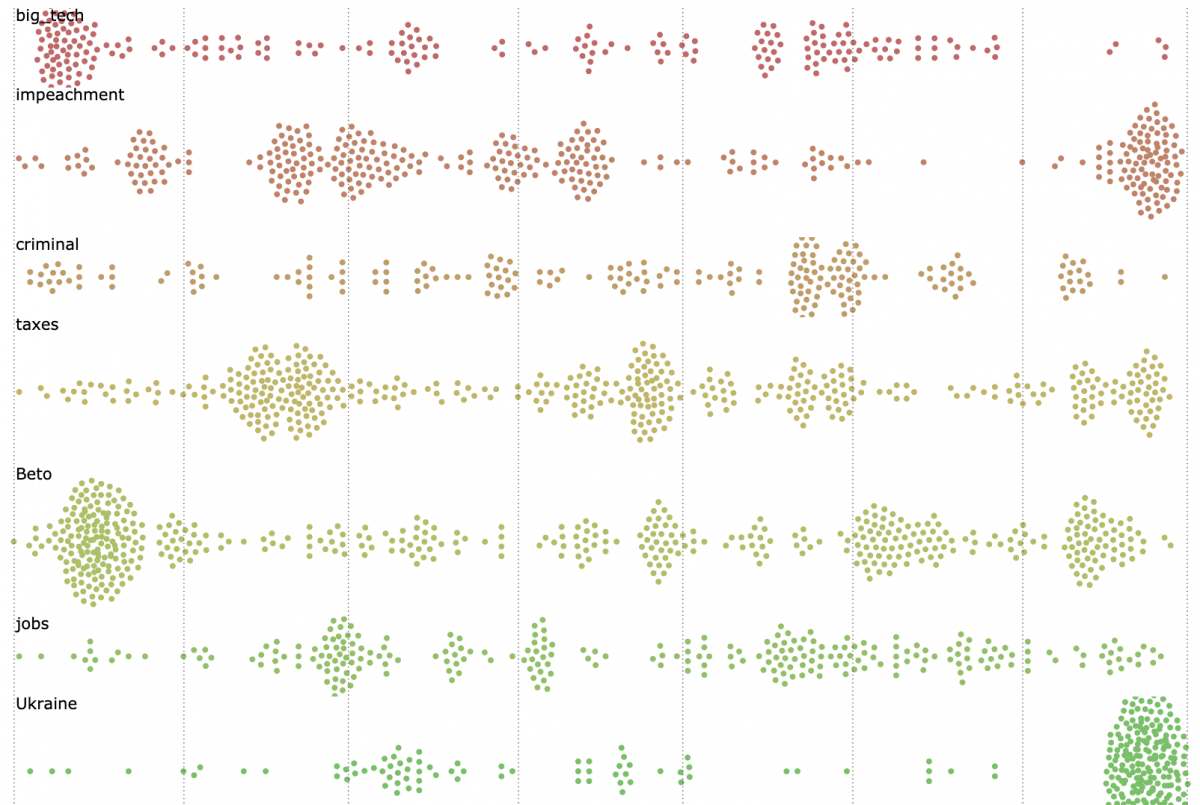
Specific candidates and key topics
Digging into each of these topics, we can calculate just how prevalent they are within coverage of a specific candidate. As one might expect, the Ukraine topic is almost exclusively associated with Biden and guns with O’Rourke. Notably, Tulsi Gabbard, Pete Buttigieg and Beto O’Rourke are most associated with immigration; Cory Booker is most associated with criminal justice; and the health care topic is most associated with Bernie Sanders.
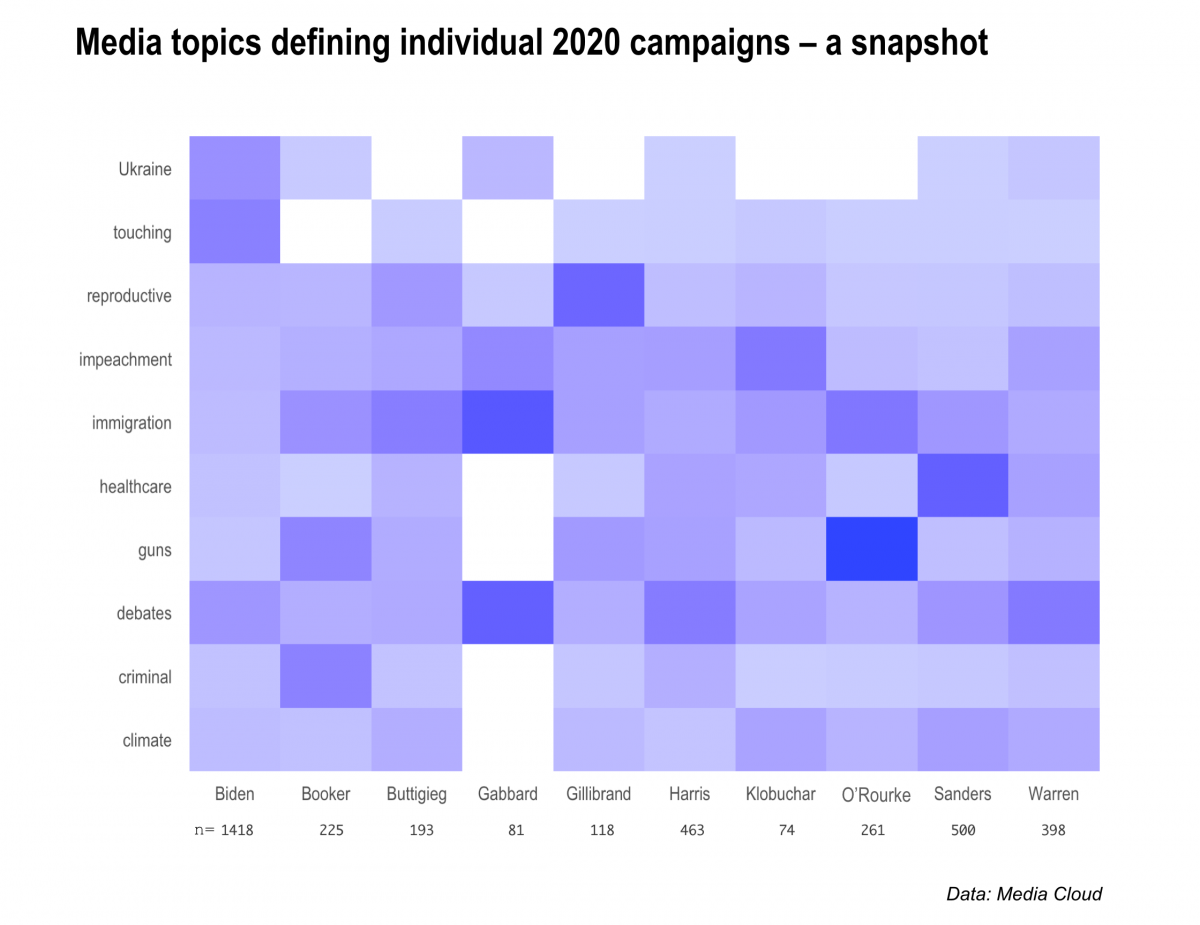
Note: The heatmap’s color scheme communicates the proportion of that topic relative to the total number of articles on that candidate, not the proportion of that candidate’s “owning” of the topic as compared to the other candidates. For instance, 27 of Gabbard’s 81 top articles, or one-third, were categorized into the debates topic while 167 of 500 Bernie Sanders articles, also one-third, were tagged in the health care topic. Thus, Gabbard and Sanders have similar color hues for those topics despite having different frequencies of coverage.
Earlier this year, New York University journalism professor Jay Rosen proposed campaign coverage built around a “citizens agenda,” which he described as identifying your audience, listening to what they believe the candidates should be focusing on, and covering the race accordingly.
“Given a chance to ask questions of the people competing for office, you can turn to the citizens agenda,” Rosen wrote on his influential blog, Press Think. “And if you need a way of declining the controversy of the day, there it is. The agenda you got by listening to voters helps you hold to mission when temptation is to ride the latest media storm.”
Complaints by candidates themselves about media coverage are a feature of nearly every campaign, but there have been some particular concerns about such rhetoric in this election cycle, given the already overheated atmosphere of media bashing in the Trump era. Still, some of the Democratic candidates and their campaigns have been pushing back on news media behavior. This would include: Sanders’s pointed critiques of the “corporate media” that covers the campaign; the Biden campaign’s anger over the deluge of media attention to his perceived “gaffes” and to Trump’s false claims about his son’s dealings in Ukraine; a lack of attention to newer, more unconventional candidates such as Andrew Yang; and scant coverage of, for example, climate change and LGBTQ issues.
Unfortunately, though, our analysis underscores that the media hasn’t broken away from poll- and controversy-driven coverage since March. Yes, quality news organizations such as The New York Times and The Washington Post have produced some excellent stories on policy, the candidates’ leadership styles, and how those politicians have met challenges over the course of their careers. But those tend to be overshadowed by day-to-day horse-race coverage – and even those news outlets aren’t immune from following the pack over the cliff.
Methods
This analysis was based on 9,854 articles published by media outlets – including CNN, NBC, The New York Times, The Washington Post, Wall Street Journal, Politico, BuzzFeed, The Blaze, Breitbart, NewsMax and Fox News – mentioning one of the following 2020 candidates in the headline: Joe Biden, Bernie Sanders, Elizabeth Warren, Kamala Harris, Pete Buttigieg, Beto O’Rourke, Cory Booker, Kirsten Gillibrand, Amy Klobuchar or Tulsi Gabbard.
After scraping full article text with Python’s newspaper3k package, we applied structural topic modeling to the text to automatically assign topics to our corpus based on word co-occurrence. Of the 60 topics assigned, we selected 10 that were highly prevalent in the dataset, bringing the total to 3,731 articles, and visualized the weekly number of articles most likely to mention those topics. More on training and evaluating this kind of model can be found here.
Full code for this analysis can be found below. Note: Our visualizations were inspired by Bloomberg’s analysis of candidate tweets and Mike Bostock’s D3 beeswarm and built with the help of RAW Graphs and Felippe Rodrigues.
library(tidytext)
library(tidyverse)
library(lubridate)
library(stm)
library(furrr)
library(future)
plan(multiprocess)
# Ingest data collected from Media Cloud
candidates301present <- read.csv("0301-2020candidates.csv", stringsAsFactors = F)
# Tokenize and filter for most prevalent words
seven_months <- candidates301present %>%
unnest_tokens(word, text) %>%
anti_join(get_stopwords()) %>%
filter(!str_detect(word, "[0-9]+")) %>%
add_count(word) %>%
filter(n > 100) %>%
select(-n)
# Create sparse Document Term Matrix
seven_months_sparse <- seven_months %>%
count(stories_id, word) %>%
cast_sparse(stories_id, word, n)
# Run STM for 60 topics
many_models <- data_frame(K = c(20,40,60)) %>% # Here you could compare a range of topics, i.e. 20, 40, 60
mutate(topic_model = future_map(K, ~stm(seven_months_sparse, K = .,
verbose = FALSE)))
heldout <- make.heldout(seven_months_sparse)
# More here https://juliasilge.com/blog/evaluating-stm/
k_result <- many_models %>%
mutate(exclusivity = map(topic_model, exclusivity),
semantic_coherence = map(topic_model, semanticCoherence, seven_months_sparse),
eval_heldout = map(topic_model, eval.heldout, heldout$missing),
residual = map(topic_model, checkResiduals, seven_months_sparse),
bound = map_dbl(topic_model, function(x) max(x$convergence$bound)),
lfact = map_dbl(topic_model, function(x) lfactorial(x$settings$dim$K)),
lbound = bound + lfact,
iterations = map_dbl(topic_model, function(x) length(x$convergence$bound)))
topic_model <- k_result %>%
filter(K == 60) %>%
pull(topic_model) %>%
.[[1]]
# Tidy the topic model and extract gamma values
td_beta <- tidy(topic_model)
td_gamma <- tidy(topic_model, matrix = "gamma",
document_names = rownames(seven_months_sparse))
# Extract top 12 terms per topic
top_terms <- td_beta %>%
arrange(beta) %>%
group_by(topic) %>%
top_n(20, beta) %>%
arrange(-beta) %>%
select(topic, term) %>%
summarise(terms = list(term)) %>%
mutate(terms = map(terms, paste, collapse = ", ")) %>%
unnest()
# Merge topics with full dataset
gamma_fix_col <- td_gamma
gamma_fix_col$stories_id <- gamma_fix_col$document
gamma_fix_col$stories_id <- as.numeric(gamma_fix_col$stories_id)
gamma_fix_col$document <- NULL
merge_gamma_w_outlet_bias <- gamma_fix_col %>%
inner_join(candidates301present_text_bias_gender, by = "stories_id")
# Add in top terms to full dataset
merge_gamma_w_outlet_bias_plus_terms <- top_terms %>%
left_join(merge_gamma_w_outlet_bias, by = "topic")
# Add tally of total articles per candidate
merge_gamma_w_tally <- merge_gamma_w_outlet_bias_plus_terms %>%
group_by(candidate) %>%
add_tally(name = "total_candidate") %>%
glimpse()
# Select 20 topics
coherent_20_topics <- merge_gamma_w_outlet_bias_plus_terms %>%
filter(gamma > 0.1) %>%
filter(topic == 17 | # biden, trump, president, ukraine, hunter, son, ukrainian, call, said, joe, giuliani, vice
topic == 30 | # debate, candidates, stage, debates, night, democratic, castro, first, former, second, two, de
topic == 40 | # immigration, border, immigrants, u.s, illegal, said, united, israel, country, states, iran, administration
topic == 42 | #biden, women, said, flores, former, joe, president, vice, uncomfortable, touching, behavior, inappropriate
topic == 25 | #gun, violence, weapons, guns, assault, control, shooting, background, mass, ban, shootings, people
topic == 4 | # president, impeachment, trump, house, bad, report, said, congress, mueller, justice, democrats, barr
topic == 9 |# bill, justice, criminal, crime, system, marijuana, reform, law, incarceration, people, mass, prison
topic == 59 | #abortion, women, amendment, rights, hyde, support, abortions, federal, said, right, roe, reproductive
topic == 34 | #medicare, health, insurance, care, private, plan, sanders, system, healthcare, said, people, government
topic == 51 | # climate, change, energy, new, green, plan, deal, fossil, fuel, clean, said, trillion
topic == 28 | # trump, president, donald, said, white, trump’s, trump's, people, house, rally, speech, racist
topic == 12 | # tax, debt, student, wealth, college, income, plan, loan, taxes, pay, americans, said
topic == 14 | # workers, union, wage, labor, pay, campaign, minimum, employees, wages, said, working, unions
topic == 41 | # gay, pence, said, president, lgbtq, faith, marriage, mike, sex, religious, vice, god
topic == 2 | # companies, facebook, google, amazon, big, said, company, break, power, data, antitrust, technology
topic == 39 |# china, trade, deal, u.s, foreign, american, chinese, policy, tariffs, world, states, government
topic == 48 | # percent, poll, biden, sanders, support, warren, voters, points, democratic, among, primary, place
topic == 13 | # o'rourke, o’rourke, beto, texas, el, former, paso, presidential, campaign, congressman, candidate, said
topic == 49 | # black, south, said, police, white, carolina, african, racial, community, american, city, color
topic == 47 # america, children, poor, make, want, kids, child, parents, family, answer, live, american
)
coherent_20_topics_frame <- coherent_20_topics %>%
mutate(frame = dplyr::case_when(
topic == "17" ~ "Ukraine",
topic == "30" ~ "debates",
topic == "40" ~ "immigration",
topic == "42" ~ "touching",
topic == "25" ~ "guns",
topic == "4" ~ "impeachment",
topic == "9" ~ "criminal",
topic == "59" ~ "reproductive",
topic == "34" ~ "healthcare",
topic == "51" ~ "climate",
topic == "28" ~ "Trump",
topic == "12" ~ "taxes",
topic == "14" ~ "jobs",
topic == "41" ~ "LGBQT",
topic == "2" ~ "big_tech",
topic == "39" ~ "China",
topic == "48" ~ "horserace",
topic == "13" ~ "Beto",
topic == "49" ~ "race",
topic == "47" ~ "poverty"
)) %>%
glimpse()
coherent_20_topics <- coherent_20_topics %>%
group_by(candidate) %>%
add_tally(name = "total_candidate") %>%
glimpse()
glimpse(coherent_20_topics)
coherent_20_topics_frame$publish_date <- as.Date(coherent_20_topics_frame$publish_date)
coherent_20_topics_frame_week <- coherent_20_topics_frame %>%
group_by(candidate, week=floor_date(publish_date, "weeks")) %>%
glimpse()
# Select variables of interest for visualization
coherent_20_topics_export <- coherent_20_topics_frame_week %>%
select(topic, frame, terms, gamma, title, url, media_name, candidate, bias, gender, publish_date, week, total_candidate)
write.csv(coherent_20_topics_export, "coherent_20_topics_export.csv")